Machine Learning in Mobile Application Development
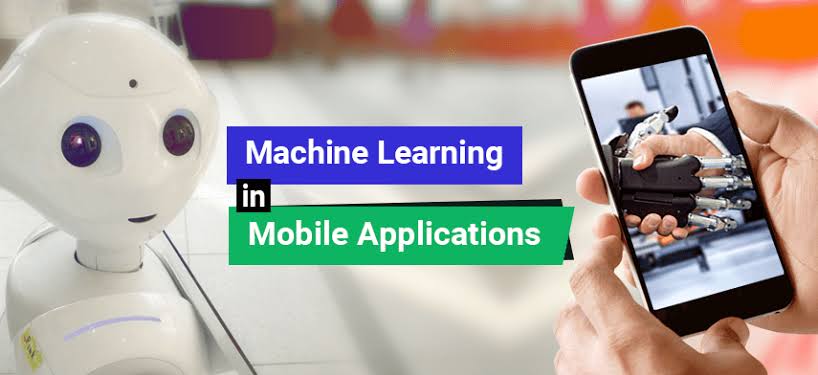
The merge of Artificial Intelligence (AI) and machine learning has transformed mobile applications to the next level. This authorizes the software to study and explore without any human interaction. Machine learning has been used in many fields. Like Facebook like how it automatically detects your face when someone uploads your picture on it. How YouTube examines your video genre and displays you on your screen is most similar to your recommendation, how your phone assistance reads your notification automatically.
Through chatbots, we can handle our entire phone. This technology is evolved that we can do a small gossip with the ap machine too. The perfect example of these features is Amazon Alexa, Apple’s Siri, and Google assistance.
Suppose you are looking to integrate machine learning into mobile applications. Then you are in the right place. This article will talk about how machine learning can be put in mobile applications and how it affects technology.
Algorithms of machine learning
Machine learning algorithms can predict targeted user behavior and searching patterns to understand the user’s suggestions and recommendations. The extensive use of this technology in mobile is in e-commerce applications. For ease of authentication, machine learning algorithms are also used in face or fingerprint recognition.
Machine learning for mobile apps
Machine learning offers innovative revolutions across the mobile application development company, and developers have to gain a lot from this. Due to technical abilities, it is most possible that the mobile application offers a smoother user interface, practices, and empowering businesses with specific features. Such as delivering goods at your precise location. Search anything on the database through a picture. This perfect example of this feature is the Google lens. Configuring the right way to detecting chronic disease.
Machine learning empowers the mobile user experience that provides an immersive and impressive experience. The practical approach of machine learning is to develop high speed for intelligent devices. In addition, machine learning in mobile applications helps developers build various, powerful apps through data filtration, parameter modification, and algorithms learning.
Artificial intelligence
Machine learning in mobile application development the storage and services can find patterns in the collecting the big data from their users. Machine learning algorithms can use unstructured data and deliver valuable perceptions into user behavior according to the data received by the machine. This will engage more clients to ask for services from the app developer that will power machine learning to improve services. For example, studying what users are interrelating with. And this is called Artificial Intelligence (AI).
Examples
The followings are some specific examples of machine learning in a mobile application.
Social media
Another example of artificial intelligence is Facebook. Facebook uses its machine learning tools to provide you a customized experience. It uses its tools in forecasting user’s behaviors. As a result, this will enable the social media giant to target relevant audiences for advertisements if you are searching for something on social media or the internet. The software will mark you as a potential customer and suggest things according to your age and preferences. And not just that the advertiser will try to recall you if you try to switch to another competitor.
Travel service
Cab services can use data from previous rides and apply machine learning to get a more accurate estimate of the time of arrival and fare of the ride based on such aspects as traffics, weather conditions, and decided routes.
Camera
Machine learning algorithms are also applicable on camera apps, both on native and downloaded on mobile phones. This will reduce the noise in the picture and provide as much as a possible clear picture. For example, for getting a clear and regular exposure picture under bright sunlight, the HDR option will automatically analyze how many multiple shots it should take to provide a better-looking image.
This is the same kind of tech used by golf simulators, where it processes captured movements to generate more insights necessary for a golfer’s improvement
Health and fitness
Such wearables, fitness bands, and smartwatches are linked with their workout apps powered by machine learning. These hardware devices track the user’s health and gather data from user behavior and then analyze it. And then, based on one’s goals and behavior, the user receives modified lifestyle assistance. Machine learning algorithms can also examine the user’s current fitness levels and eating habits to provide a personalized fitness plan. For example, how much exercise to do for burning that number of calories.
This technology is a future move in another category nowadays the machine learning can do bioelectrical impedance analysis BIA from the wearable. The process uses the weak electric current to find the amount of water storage in the body. This will help your body weight reflect the number of muscles, fat, and water amount in tissues. And by measuring this over time, you can adjust your diet and activity level.
These wearables connect with mobile applications measure your potential by tracking your heartbeat and oxygen level. This will help you from heart illness.
Top machine learning mobile application example
The way users interact with the devices and algorithms will transform and bring unique machine learning to our daily mobile apps. The list below is the best examples of mobile applications that use machine learning.
Snapchat
The Looksery Ukrainian started this app, and later on, Snapchat acquired it for 150 million dollars. This application uses the supervision of machine learning algorithms for computer vision in the beginning. But now Snapchat turned it into a prototype camera with the help of machine learning algorithms. Now, this app finds faces with the help of a camera. The application adds fun elements to the face like funky glasses, funny-looking ears, dogs, and hats. These are the filters the artificial intelligence (AI) accurately detects and adds filters to your face. These filters also move with the movement of the face. These filters also have some other fun essentials that will react with the moment of the face. These filters have three-axis views.
And last but not least, Snapchat also offers geofilters. This algorithm has some graphical overlay that is available when a user is in an accurate geographical location. For example, it adds the city or destination’s famous place’s picture on the screen in the form of a sticker.
Tinder
Tinder is a dating app that finds your perfect match through pictures. It uses machine learning algorithms to find a precise match according to the age and distance range you enter in the app. This app randomly shows one user’s photos to another user. Machine learning analyzes how many left and right swipes does one picture received. As a result, tinder learns which image is more attractive and which is less appealing. This algorithm will help users with which image they should place on the 1st.
Spotify
Spotify is the world’s most famous audio streaming mobile application. Spotify forces three machine learning algorithms to provide customized music recommendations to users in the daily discovery section and increase engagements.
- The first type of Spotify uses an algorithm of collaborative filtering. This algorithm delivers users with modified music references by comparing songs users have listened to with a playlist created by multiple users. Then, the algorithm searches those playlists to look at other songs from the user’s playlist and recommend them.
- In the second type, the machine learning algorithms use natural language. In the process, the software reads song lyrics, blog posts, articles about particular music artists, and news blogs about songs and musicians on the internet. Then, based on this data, the algorithm classifies into “cultural vector” and “top terms” and recommends songs with similar meanings.
- The audio model is the third type of machine learning algorithm. In this type, machine learning tools examine the information from raw audio tracks, catalogs songs, and suggest other songs with similar songs, famous among other users.
Netflix
The number no one live streaming platform Netflix uses reversion and machine learning algorithms too. It has made more exact and personalized recommendations by using linear and logistic regression and more such algorithms. The Netflix application shows varied content, actors, timespan, user’s and critic’s reviews, years, and much more to suggest to their audience. And all this data goes into machine learning algorithms.
The machine learning process in Netflix goes through its user’s actions that track their behavior of using the application. It monitors what kind of TV shows and series the user mostly watches and what kind of reviews the user provides on the app. And machine learning algorithms explain these user behaviors to offer exceptionally customized content.
Final Thoughts
Machine learning decreases the gap between understanding user behavior and utilizing it to create a personalized solution. Machine learning depends on continuously learning based on their user’s daily activities. This progression helps to develop an adaptive mobile application that can help in providing an ideal user experience. Furthermore, machine learning enables intelligent platforms to support you in creating and training the required analytical models.